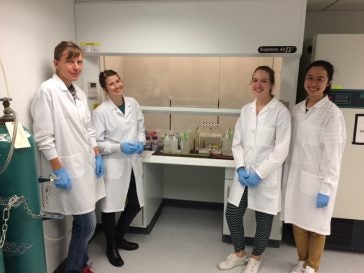
Private wells contaminated by PFAS can be a substantial source of PFAS exposure but monitoring initiatives are costly, time consuming, and tend to be conducted on a more ad hoc basis. STEEP researchers from the Sunderland laboratory group developed a statistical approach to help prioritize sampling locations for private wells by analyzing the hydrological proximity of wells to different PFAS sources as well as environmental characteristics likely to affect PFAS transport. This machine learning model was used to interpret data collected between 2014 and 2017 by the New Hampshire Department of Environmental Services (NHDES) on concentrations of 35 PFAS in 3700 groundwater samples from over 2300 unique wells. The project team divided these data into training (70%) and testing (30%) datasets. Performance was tested for two categorical models, which predict whether a well is likely to have detectable PFAS (logistic regression, classification random forest), and one continuous model, which predicts a discrete concentration (regression random forest). The continuous model explained 2-52% of the variance in private well PFAS concentrations, suggesting more data are needed to accurately predict private well concentrations. However, the categorical models performed better with an accuracy of 66-82% for the logistic regression and 75-83% for the classification random forest model. Significant predictors in the categorical models included PFAS point sources such as plastics and rubber, printing, and textile industries, and factors affecting PFAS transport such as groundwater recharge, precipitation, soil clay content, and infiltration capacity. Incidence of missed PFAS detections (false negatives) averaged 17% across the five PFAS modeled, suggesting suitability of the approach for prioritizing sampling and identifying vulnerable areas.