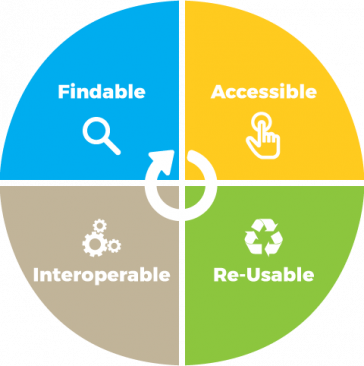
In recent years, there has been a push to make data “FAIR” — Findable, Accessible, Interoperable, and Reusable. These principles promote the reuse of scholarly data and make data accessible for machine learning and use in meta-analysis studies. To incorporate these principles, and with additional funding from NIEHS, STEEP has teamed up with Harrison Dekker, a data science librarian at URI, and graduate research assistant Yana Hrytsenko to assess and improve data workflows and integrate FAIR practices. Training will emphasize data management, code management, project organization, and communication.
One goal for STEEP is to be able to systematically compare the effects of PFAS on different animals (e.g., fish, mice, and birds) in both field and laboratory settings. Generated data need to be understood by the different research groups, standardized (harmonized) in order to be read by machine learning algorithms, and organized in a way that allows the final enhanced data to be easily findable and accessible. Data will be formatted in a way that explains the methods by which PFAS were measured and how results were generated, so they are easily understood by future users. For example, STEEP is currently working to integrate field data generated by three university laboratories (URI, Harvard, and UC-Denver) and USGS. Applying FAIR principles to these data will more readily provide understanding of the bioaccumulation of PFAS in fish exposed to contaminated water and how passive samplers can help understand the bioaccumulation.
This data integration effort relates to STEEP’s mission to holistically understand exposures and effects of PFAS.