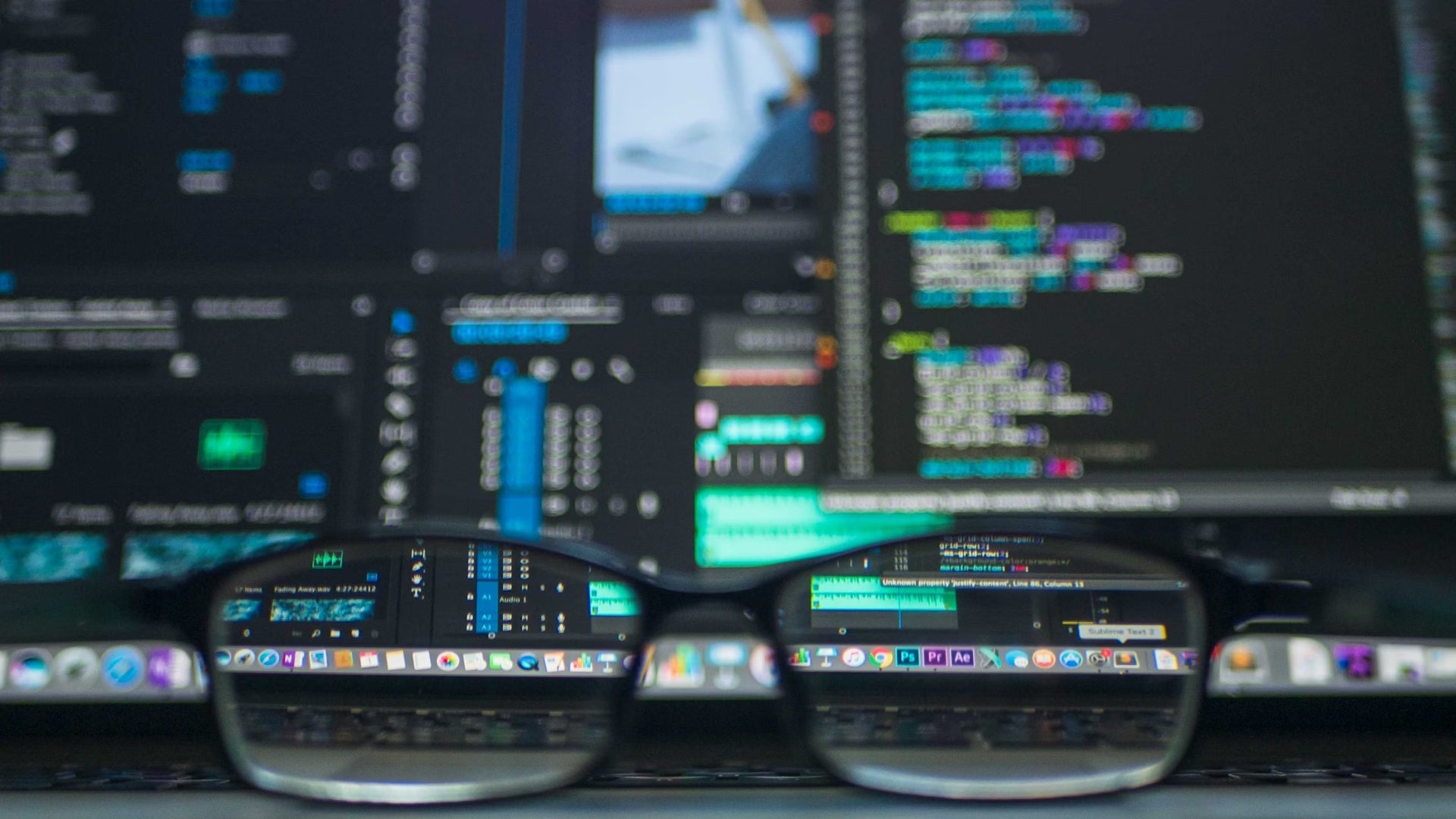
Machine Learning Co-Exploration
Leonardo Macelloni (USM) and Mike Jakuba (WHOI)
Photo by Unsplash
Overview
Presently, deep ocean exploration and mapping using AUVs is efficient and convenient in terms of logistics and costs, however, this approach suffers from the major technological impediment that the bulk of the data collected, both in the form of multibeam survey and or seafloor images cannot be analyzed in real-time. There is little “conversation” between operators and subsea platforms via the narrow bandwidth of acoustic communication so redirecting or changing AUV mission plans to interrogate “promising” targets, narrow the area of research to increase the resolution, or even change the mapping tools can be done only after the data are downloaded, analyzed and interpreted a posteriori.
This project addresses a key challenge in bringing advanced autonomy to the deep sea. Opportunities for experimentation are few and expensive. CoEx enables developer access to vehicle processes in the same way that it facilitates operator and scientist access to vehicle data, allowing developers to monitor and alter algorithm performance in real-time, and to gradually grant subsea autonomy greater control over the vehicle (e.g. by initially requiring topside validation of proposed mission alterations). This project is a joint effort between USM, in partnership with the University of Louisiana Lafayette, the Center for Computational Geosciences, and two groups at WHOI, the Girdhar group and the Jakuba group. The project will be organized into three complementary components. Two components will be devoted to the development of ML algorithms for in-situ seabed exploration and a third component consisting of CoEx hardware and software development to facilitate the implementation, testing, and operational transition of the algorithms during a field program.