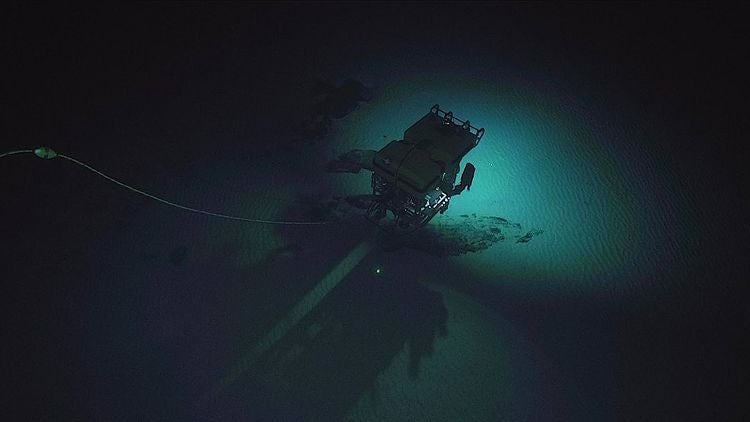
Machine Learning Video
Adam Soule (URI)
Photo by OET
Overview
Deep-sea video is one of the most important data sources in deep-sea science, but also an extreme challenge for data usage and archiving due to the very large data volumes produced. For general use, underwater dive videos can be sparse, with only a few high-value clips interspersed with hours of video relevant only to specific domains. The process of condensing such high-volume data can be time-consuming as human annotators must manually clip videos to identify highlights. To help, OECI-supported researchers and graduate students developed ROVIA, a portable and field-deployable CNN (Convolutional Neural Network) model to identify potential biological, geological, and operational highlights from long-dive videos. This automated video highlight generator provides increased efficiency in condensing deep-sea video to aid in archiving and enhance the utilization of clips for scientific and educational purposes.
This project is available on GitHub
view repository